






12:37 pm

February 4, 2015

A self-organizing map (SOM) or self-organizing feature map (SOFM) is a type of artificial neural network (ANN) that is trained using unsupervised learning to produce a low-dimensional (typically two-dimensional), discretized representation of the input space of the training samples, called a map. Self-organizing maps are different from other artificial neural networks in the sense that they use a neighborhood function to preserve the topological properties of the input space.


A self-organizing map showing U.S. Congressvoting patterns visualized in Synapse. The first two boxes show clustering and distances while the remaining ones show the component planes. Red means a yes vote while blue means a no vote in the component planes (except the party component where red is Republican and blue is Democratic).
This makes SOMs useful for visualizing low-dimensional views of high-dimensional data, akin to multidimensional scaling. The artificial neural network introduced by the Finnish professor Teuvo Kohonen in the 1980s is sometimes called a Kohonen map or network.[1][2] The Kohonen net is a computationally convenient abstraction building on work on biologically neural models from the 1970s[3] and morphogenesis models dating back to Alan Turing in the 1950s[4]
Like most artificial neural networks, SOMs operate in two modes: training and mapping. “Training” builds the map using input examples (acompetitive process, also called vector quantization), while “mapping” automatically classifies a new input vector.
The following users say thank you to Hema Harisan for this useful post:
Costin3:54 am
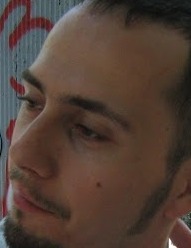
Premium Member
ByPassPostChallenge
January 26, 2014

In practice, 3D detectors are parts of sophisticated apps, which aren`t quite affordable, aimed to discover metal or dangerous substances such as mercury into a certain environment
The area is still in pioneer phase, but it seems that it delivers encouraging results
Most Users Ever Online: 1255
Currently Online:
7 Guest(s)
Currently Browsing this Page:
1 Guest(s)

Top Posters:
Costin: 1019
23and14and20: 421
Raisaa: 401
Krisstoffer: 398
Jackson: 277
Cellnut: 192
Talla: 166
thethunderchild: 148
Paul B: 130
jangwl: 121
Member Stats:
Guest Posters: 0
Members: 52196
Moderators: 4
Admins: 2
Forum Stats:
Groups: 7
Forums: 224
Topics: 1710
Posts: 5839
Newest Members:
Rigoberto AmessModerators: scott: 3, Compudoctores: 0, ctech: 0, Help Desk: 0
Administrators: ctsuperadmin: 3, ctsupportadmin: 3